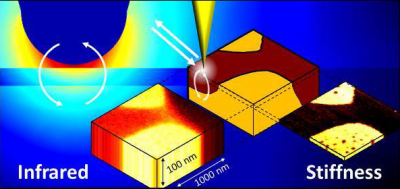
Researchers from the Nanooptics Group at CIC nanoGUNE (San Sebastian, Spain) have shown that nanoscale infrared imaging, which is currently used as a surface-sensitive technique, can be employed for chemical nanoidentification of materials that are located up to 100 nm below the surface. The results further show that the infrared signatures of thin surface layers differ from that of sub-surface layers of the same material, which can be exploited to distinguish the two cases. The findings, published in Nature Communications (doi: http://doi.org/d4vb), push the technique one important step further to quantitative chemometrics at the nanoscale in three dimensions.
Optical spectroscopy with infrared light, such as Fourier transform infrared (FT-IR) spectroscopy, allows for chemical identification of organic and inorganic materials. The smallest objects which can be distinguished with conventional FT-IR microscopes have sizes on the micrometre-scale. In nano-FT-IR (which is based on near-field optical microscopy), IR light is scattered at a sharp metallised tip of a scanning-probe microscope. The tip is scanned across the surface of a sample of interest and the spectra of scattered light are recorded using FT detection principles. Recording of the tip-scattered light yields the sample’s IR spectral properties and thus the chemical composition of an area located directly below the tip apex. Because the tip is scanned across the sample surface, nano-FT-IR is typically considered to be a surface-characterisation technique.
Importantly though, the IR light that is nano-focussed by the tip does not only probe a nanometric area below the tip, but in fact probes a nanometric volume below the tip. Now the researchers at CIC nanoGUNE showed that spectral signatures of materials located below the sample surface can be detected and chemically identified up to a depth of 100 nm. Furthermore, the researchers showed that nano-FT-IR signals from thin surface layers differ from that of sub-surface layers of the same material, which can be exploited for determination of the materials distribution within the sample. Remarkably, surface layers and sub-surface layers can be distinguished directly from experimental data without involving time-consuming modelling.